By MedGenome Scientific Affairs
Introduction
Colorectal cancer (CRC) stands as the third most prevalent cancer and the second leading cause of cancer-related deaths in the US. It is projected that in 2024, there will be around 106,590 new cases of colon cancer and 46,220 new cases of rectal cancer. CRC incidence is notably higher among African Americans and lowest in Asian Americans/Pacific Islanders. The five-year relative survival rate for localized CRC is estimated to be 91%, contrasting with a 14% rate for metastatic disease. Mortality rates among older adults have seen a decline in recent years owing to factors such as the implementation of screening programs, advancements in imaging technology for precise staging, improvements in surgical procedures, and the development of new treatment modalities. Nevertheless, concerning trends reveal a 1% annual increase in CRC mortality rates among individuals under 55 since the mid-2000s1,2.
Risk factors
Risk factors for CRC include genetic predisposition, environmental factors, and lifestyle behaviors such as obesity, smoking, and unhealthy diet. Long-standing ulcerative colitis and Crohn’s disease increase CRC risk. Other factors include family history of cancer, colon polyps, diabetes mellitus, and cholecystectomy. Additionally, gut microbiome composition, age, gender, race, and socioeconomic status influence CRC risk2.
Molecular pathways associated with CRC carcinogenesis
Approximately 75–80% of CRCs are sporadic, originating from the accumulation of genetic and epigenetic alterations within specific molecular pathways. These pathways play a crucial role in regulating cell growth, differentiation, and survival. This intricate process of carcinogenesis, known as the adenoma–carcinoma sequence, entails mutations in at least 15 cancer-related genes. From a molecular point of view, CRC is highly heterogeneous and this can be ascribed to three major molecular pathways. The most prevalent pathway, accounting for 85% of sporadic CRCs, is chromosomal instability (CIN). CIN is a hallmark of genomic instability and is characterized by gain and loss of large chromosomal segments, leading to gene copy number variations, frequent loss of heterozygosity (LOH) at specific gene loci and chromosomal rearrangements. These alterations often involve mutations in specific oncogenes such as BRAF, KRAS, PIK3CA or tumor suppressor genes such as APC, SMAD4 and p53, which regulate cell proliferation and play crucial roles in CRC initiation and progression pathways. The second pathway is the CpG island methylator phenotype (CIMP), evident in 15% of CRC cases. This pathway is characterized by the hypermethylation of CpG islands in their promoter regions resulting in the epigenetic silencing of the adjacent genes. The third one is microsatellite instability (MSI), accounting for about ~13–16% of sporadic cases and is often associated with hereditary forms of the disease, such as Lynch syndrome. This pathway results from defects in the DNA mismatch repair (MMR) genes responsible for correcting errors during DNA replication3,4.
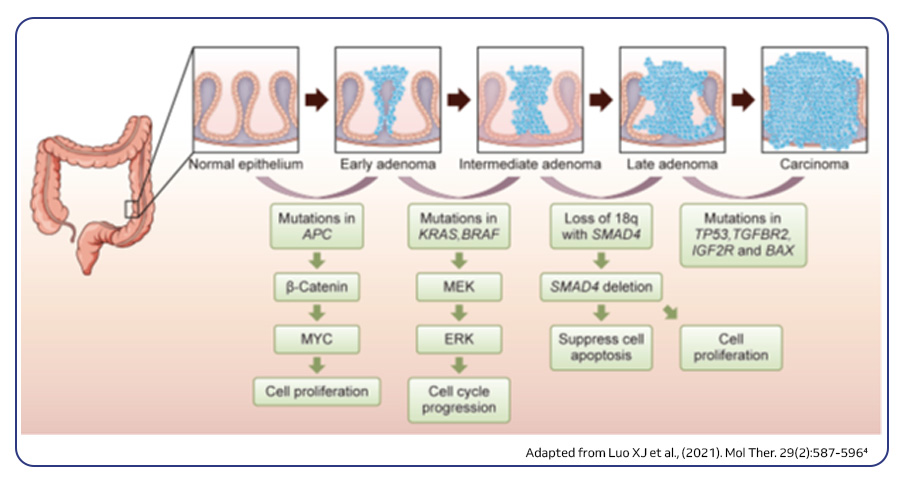
Colorectal cancer: from genomic profiling to precision medicine
Genomic insights
Next-generation sequencing (NGS) technologies have revolutionized the understanding of CRC by facilitating comprehensive genomic profiling of tumors. In the past decade, extensive sequencing investigations have explored the genetic foundations of CRC, revealing significant pathways involved in its development, such as WNT, RAS-MAPK, PI3K, TGF-β, P53, and DNA mismatch repair pathways. NGS technology, utilized by global consortia like The Cancer Genome Atlas (TCGA) Research Network, adopted a comprehensive approach, examining exome sequences and DNA copy numbers, clarifying epigenetic modifications, and delineating the role of microRNA in human cancers, including CRC. These studies provided fundamental genetic insights and identified numerous new theranostic and prognostic molecular biomarkers, prompting further investigation and integration into clinical trials. These findings highlighted the genetic diversity of colorectal cancer, challenging its prior classification as a histopathologically homogeneous disease5.
Recently, single-cell RNA sequencing has effectively enhanced current molecular classifications of CRC by identifying unique sub-clones within previously identified subtypes through bulk transcriptomics, providing potential prognostic insights. Moreover, single-cell multi-omics approaches have been employed to monitor transcriptomic and epigenomic alterations in CRC, as well as to identify clinically relevant cell sub-clones linked to cancer progression and metastasis5.
Immunotherapy for metastatic CRC
Immunotherapy, particularly immune checkpoint inhibitors, has emerged as a promising treatment modality in CRC. Currently, immunotherapy in CRC treatment is primarily used for patients with metastatic disease and whose tumors are mismatch repair deficient (dMMR) or microsatellite instability-high (MSI-H). These tumors accumulate a higher number of mutations, making them more easily recognized and targeted by the immune system. Genomic research is focused on identifying biomarkers, such as tumor mutational burden (TMB) and immune-related gene expression profiles, that can predict response to immunotherapy. Additionally, researchers are investigating strategies to enhance the efficacy of immunotherapy in CRC, including combination approaches with other targeted therapies or chemotherapy.
Role of precision medicine
The concept of precision medicine, which involves tailoring treatment strategies to individual patients based on their unique genetic makeup and tumor characteristics, is gaining traction in CRC research. Although targeted therapies have improved outcomes for some patients, predictive models incorporating genetic and environmental factors for risk assessment and personalized screening are emerging but need validation across various populations. Precision medicine approaches aim to maximize treatment efficacy while minimizing adverse effects.
Liquid biopsies, a minimally invasive approach
Liquid biopsy techniques, such as circulating tumor DNA (ctDNA) analysis and circulating tumor cell (CTC) enumeration, are being increasingly utilized in CRC research and clinical practice. Liquid biopsies offer a minimally invasive method for monitoring disease progression, detecting minimal residual disease, and identifying treatment-resistant mutations. In addition to plasma cfDNA levels, which have conventionally been associated with tumor burden, sequencing cfDNA has demonstrated the ability to recapitulate the mutational profile of the primary tumor. They have the potential to revolutionize cancer diagnosis, monitoring, and treatment response assessment.
Characterization of tumor microenvironment
The tumor microenvironment (TME) plays a crucial role in CRC progression and response to therapy. Genomic research is investigating the complex interactions between tumor cells, immune cells, stromal cells, and the extracellular matrix within the TME, including the gut microbiota. Understanding these interactions may lead to the development of novel therapeutic approaches targeting the TME, such as immune-modulating agents and stromal-targeting therapies.
Molecular target | Targeted therapy | Mechanism of action |
---|---|---|
VEGF | Bevacizumab, Ramucirumab, Ziv-aflibercept and Fruquintinib | Inhibits angiogenesis mediated through VEGF pathway |
EGFR | Cetuximab and Panitumumab | Binds to external domain of EGFR receptor and prevents its activation |
BRAF | Encorafenib | Targets key enzymes in the MAPK signaling pathway |
HER2 | Trastuzumab, Pertuzumab and Lapatinib | Binds to extracellular domain of HER2 (Trastuzumab), inhibits the heterodimerization of HER2 (Pertuzumab) and disrupts the downstream signaling pathways activated by HER2 (Lapatinib) |
NTRK | Larotrectinib and Entrectinib | Inhibits the tropomyosin-related kinase (TRK) receptor domains found in TRKA, TRKB, and TRKC proteins, resulting in reduced cellular proliferation |
RET | Selpercatinib | Inhibits RET kinase through ATP competitive mechanism |
KRAS | Adagrasib and Sotorasib | Binds to and stabilizes RAS in its GDP-bound state, leading to decreased signal transduction, particularly through the RAF-MEK-ERK/MAP pathway |
Immune checkpoint inhibitors | ||
MSI or Deficient MMR | Pembrolizumab, Nivolumab and Dostarlimb | Binds to PD-1, a receptor expressed on activated T cells, inhibiting its activation by ligands resulting in the activation of T-cell-mediated immune responses against tumor cells |
MSI or Deficient MMR | Ipilimumab | Inhibits CTLA-4 leading to T cell activation |
Conclusions
A concerted global research endeavor is currently underway to advance treatments for colorectal cancer. This research encompasses a broad range of activities, from fundamental investigations aimed at unraveling the biological intricacies of CRC to inquiries into the societal factors influencing cancer risk. By adopting a multifaceted approach, we aspire to a future where CRC is not only prevented and detected at its earliest stages but also treated with personalized, efficient, and accessible strategies, ultimately enhancing the quality of life for patients worldwide.
MedGenome offerings
At MedGenome, we provide advanced NGS solutions with optimized workflows and protocols. As a 10x certified service provider, we also offer state-of-the-art single-cell sequencing solutions. Our robust in-house bioinformatics platform is tailored to transform raw data into actionable insights, delivering publication-ready, high-quality figures, and detailed reports across a range of NGS data types.
Please reach out to us at research@medgenome.com to get in touch with our expert scientific team for any queries and additional details.
To know more about our unique cancer genomics solutions and services please click on the following links: Whole genome and whole exome sequencing, RNA Sequencing, Single cell sequencing, Immune profiling and Epigenetic profiling
References
-
- https://www.aacr.org/patients-caregivers/cancer/colorectal-cancer/
- https://www.cancer.org/cancer/types/colon-rectal-cancer/about.html
- Huang Z and Yang M. (2022). Molecular Network of Colorectal Cancer and Current Therapeutic Options. Front Oncol. 12:852927.
- Luo XJ, Zhao Q, Liu J, Zheng JB, Qiu MZ, Ju HQ and Xu RH. (2021). Novel Genetic and Epigenetic Biomarkers of Prognostic and Predictive Significance in Stage II/III Colorectal Cancer. Mol Ther. 29(2):587-596.
- Kyrochristos ID, Ziogas DE, Goussia A, Glantzounis GK and Roukos DH (2019). Bulk and Single-Cell Next-Generation Sequencing: Individualizing Treatment for Colorectal Cancer. Cancers (Basel). 11(11):1809.
#Colorectal cancer, #Next generation sequencing, #Genomic instability, #Genomic profiling, #Exome sequencing, #Single cell sequencing, #10x Chromium, #Targeted therapy, #Precision medicine, #Immunotherapy, #Immune checkpoint inhibitors, #Tumor microenvironment